CLIMATE CHANGE MODELLING: PREDICTION OF AIR QUALITY INDEX IN ALMATY CITY
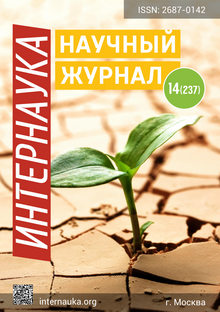
CLIMATE CHANGE MODELLING: PREDICTION OF AIR QUALITY INDEX IN ALMATY CITY
Elvira Apsalyamova
Master of Faculty of Information Technology, Kazakh-British Technical University,
Kazakhstan, Almaty
ABSTRACT
Air quality topic is a big concern in the modern fast-growing world. Good air quality is an essential requirement for healthy life of people, animals, natural resources. Air quality is mainly affected by greenhouse emissions generated by human activities like heavy traffic, food consumption, manufacturing, and construction, etc. Poor air quality leads to global climate change from long term perspective. This paper investigates the main causes of air pollution. There are many works that raise this important issue, but the peculiarity of this article is that the air quality in the city of Almaty is considered here. This work aims primarily at predicting air quality index for the next 3 days, so that it would be possible to classify whether this index is at risk. For a more accurate prediction, the paper considers several Fuzzy algorithms as Fuzzy logic, Fuzzy Time Series and Fuzzy Neural Network.
Keywords: Climate change, air quality index, fuzzy logic.
Introduction
Good air quality is a basic requirement for preserving the exquisite balance of life on earth for humans, plants, animals, and natural resources. Air pollution affects human health and the natural environment in various ways, from direct and immediate effect like health issues and various decease to the slow and gradual destruction of the body’s life-support systems. This impact of pollutants on the human body can lead to the most serious consequences. For example, sulfur dioxide in combination with atmospheric moisture forms to sulfuric acid, which destroys lung tissue of humans and animals. Particularly, sulfur dioxide is very dangerous, when it precipitates on dust particles and then penetrates deep into the respiratory tract. Dust containing silicon dioxide (SiO2) can initiate different lung diseases. Nitrogen oxides irritate and can also destroy the mucous membranes (eyes, lungs). Especially, they are dangerous in the air with sulfur dioxide and other toxic compounds. The effect of carbon monoxide (CO) on the human body is widely known: the general weakness, dizziness, nausea, drowsiness, loss of consciousness. The smaller the size of the particle, the more dangerous effect it brings to human health. Due to their small size, they can penetrate in the lymph nodes, linger in the lung alveoli, clog mucous membranes. PM2.5 concentration is one of the measures used for evaluation of air quality.
PM2.5 is particulate matter with the size lower than 2.5 microns. The size of PM 2.5 is approximately 30 times smaller than the average width of the human hair as it can be seen on the Figure 1. This tiny size makes them almost impossible to monitor. PM 2.5 comes from plenty of sources like wildfires, power plants and industrial processes or they can be created by combination of different chemicals in the air.
Due to their tiny size, these particles can get around the body’s defenses against unwanted intruders. PM 2.5 particles together with other dangerous contaminants can float from the nose to the brain bypassing blood-brain barrier. Thus, it can lead to various brain diseases and other health issues.
Figure 1. Size comparisons for PM particles
An air quality index (AQI) is a measure that shows the current or forecast air pollution. It is mainly used by government agencies to interpret the level of air pollution to the public. Air quality standards and indices are differed from country to country. The air quality index is calculated by linear function where inputs are the pollutant concentration. Usually, the ranges of air quality categories start with good level (0-50) and ends up with hazardous level (more than 300) as it is shown in the Figure 2.
Figure 2. AQI categories
There are plenty of discussions about the main reasons for the high PM2.5 level in Almaty city. The main assumptions are heavy traffic in the city and high emissions of CO2 produced by the largest coal-fired combined heat and power plant in the city is CHP-2 (located at the north-west) and burning coal and other materials during the heating season. There are several measures which we can take to make air quality better not only for us but even for the coming generations.
The most important environmental consequences of global atmospheric pollution include:
1. possible climate warming (” greenhouse effect”);
2. disruption of the ozone layer;
3. acid rain.
This work aims to build an air quality prediction model to define the main predictors that affect air quality. This can help the government to define the areas for improvement. The main challenge I faced in my work is to collect data for modelling. First, sensors for PM 2.5 measurement have been installed in Almaty from 2017 year only. Also, the data coming from sensors is not available for all sensors for all days.
Literature Review
Plenty of research papers have been reviewed the topic of climate change or air pollution. Some papers aim to predict air quality in an area for the next day, based on the past data of air pollutant emissions and meteorological and atmospheric conditions, and other geographical features. Other papers consider models for long term prediction, for example, how the global mean temperature increase is distributed by 2100 year.
According to the paper [1] the fuzzy logic models can be used to determinate the global temperature increases by 1, 2, 3 and 4 °C for 2100. The main idea of this work is to use fuzzy sets to assign uncertainties for regional temperature increase and precipitation change percentage.
The paper [2] has been reviewed the problem of low accuracy of existing air prediction models, Authors have been designed a denoising autoencoder deep network model based on long short-term memory networks. The main idea was to apply noise reduction processing on monitoring data to improve the accuracy of air quality predictions.
Also, some papers are come up with an algorithm to infer the air quality indications throughout the city, for example, paper [3] built a model for Shenyang city, China. In this paper, a random forest approach was used to predict air quality index. The next data collected by urban sensing was used as an input in random forest: meteorology data, traffic and point of interest meaning the popularity of the place across the citizens and building distributions. The performance of this model has been compared with three other algorithms Naïve Bayes, Logistic Regression, Single Decision Tree and Artificial Neural Network and the results have been shown that random forest approach achieves better prediction precision.
Moreover, research [4] concluded that there is a severe air quality degradation in Almaty, which is confirmed by both the national air quality monitoring network and Airkaz independent monitoring network.
The paper [5] made the analysis of the latest data on the study of the impact of solid and gaseous components of emissions from vehicles on human health and the environment of a modern city. Also, the most dangerous components of exhaust are identified, and the new types of vehicle fuel has been analyzed in terms of its impact on the reduction of the harmful effects of exhaust on the ecology of the city and human health.
The work [6] aims to analyze the role of machine learning techniques in enriching the prediction performance. The result of the work shows that machine learning techniques are mainly popular in Europe and America continents. Also, it shows that pollution estimation works are generally conducted by using ensemble learning and linear regression-based approaches, whereas neural networks and support vector machines-based algorithms are usually used in papers with forecasting tasks.
The paper [7] reviewed several ideas: setting the value of hyper-parameters for support vector machines regression, selection of insensitive zone, investigation of the importance of Vapnik’s loss funciton.
Complexity and accuracy of climate models has been significantly improved because of the availability of different new data sources. If only atmospheric data has been available in 1970, then plenty of new data sources like Land Surface, Ocean and Sea Ice, Carbon Cycle, etc. are available now as it is shown in the Figure 3.
Figure 3. New data sources for Climate data set
However, all these works have been prepared for air quality analysis mainly in China. China has PM2.5 sensors in more than 1000 cities and plenty of data collected from sensors. There is lack of air quality research for Almaty city mainly because pm2.5 data has been started to be widely measured only recently.
Detailed algorithm and methodology
In this section, I will give the information of every step of this work and will discuss all models I have used to predict the PM2.5 level.
The figure bellow represents the algorithms used in this work. (4).
Figure 4. Algorithms used in the project
Firstly, I started by gathering the needed data for the research. Dataset collection began with obtaining information from the website [8]. There are 18 active sensors located in the city of Almaty and on the territory of the Almaty region. Sensors can be considered as sensors for the recognition of harmful gases, heavy metals, and suspended particles. All of them were scattered throughout the city to give a clear picture of the situation with the polluted air in the city. The location of the sensors was uniform: they are located both in the city center and on the outskirts. The average data of PM2.5 of all active censors has been calculated for the period from 01.01.2021 to 16.12.2021. It should be mentioned that there were some gaps in the period on several sensors. Also, I have gathered information about historical data of the daily temperature, atmospheric pressure, and speed of the wind for the same period.
After the dataset was ready, I trained the models. For the main task of my work, it was decided to take the Fuzzy algorithms. For better conduction of the results and for accurate prediction various Fuzzy models were used.
Fuzzy logic is a form of logic when the truth value of variables can be different value in the range from 0 to 1. In contrast, the values of variables in Boolean logic can be either 0 or 1. The main concept of fuzzy logic is to find partial truth from the range between completely false and completely trues.
The first step of fuzzy logic is the fuzzification. Fuzzification uses degree of membership within the interval [0,1] to assign the numerical input to fuzzy sets. The value of the degree of membership equals to 0 means that the value does not belong to the given fuzzy set. In opposite, degree of membership 1 stands for the statement that the value completely belongs within the fuzzy set. Any other value in the range between 0 and 1 shows the degree of uncertainty that the value belongs to the set. The fuzzy sets are usually represented in the linguistically form. Thus, the input value to fuzzy sets, we can be described by words. Then it is necessary to create fuzzy rules in form of IF-THEN rules, where input or computed truth values is mapped to desired output truth values. The next step is defuzzification. The goal of this step it to convert fuzzy truth values into continuous variable. The figure 5 shows how input variables have been categorized by degree of membership function, how rules have been defined and how defuzzification has been applied on the output.
Figure 5. Fuzzy Logic
Time series are datasets that contains data of the behavior of one (or more) random variable over the timeline. The main characteristic of time series is that consequence of this variable is not independent of each other. The order of these values can be considered during analysis of their appearance. The main idea of time series models is to predict future values based on the history of the same series. In this work I used Chen model as it is shown on the Figure 6. Chen proposed the high-order Fuzzy Time Series model, focusing on three main issues: fuzzification, fuzzy logic relations, and defuzzification.[9]
Figure 6. Fuzzy Time Series
A fuzzy neural network or neuro-fuzzy system is a learning machine that finds the parameters of a fuzzy system (i.e., fuzzy sets, fuzzy rules) by exploiting approximation techniques from neural networks. Table 1 shows the difference between Neural Network and Fuzzy Systems.
Table 1.
Comparison of Neural Network and Fuzzy Systems
Neural network |
Fuzzy Systems |
no mathematical model necessary |
no mathematical model necessary |
learning from scratch |
apriori knowledge essential |
several learning algorithms |
not capable to learn |
black-box behavior |
simple interpretation and implementation |
Figure 7 illustrates the parameters of Neural Network used in the modelling.
Figure 7. Fuzzy neural network
The best results on the testing dataset were shown by Fuzzy Neural Network.
However, the first model, where fuzzy logic is used, also has good results, and the model is the easiest to understand.
Prediction
Using forecast whether data for the next 3 days 20th, 21st and 22nd of December, I have calculated AQI for these dates.
Figure 8. Prediction of the air quality index
The actual results were very close to the results of Fuzzy logic.
Limitations, Recommendations and Conclusion
The conducted research has raised an important ecological issue of the quality of the air. The overall goal was to predict the air quality index for the next 3 days in Almaty city. During the research different fuzzy algorithms have been used.
The outcome of our research confirms that this model can be used for the prediction of Air Quality Index for the next 3 days in Almaty from pm2.5 perspective.
The results of the constructed models can be improved by adding new features related to auto traffics, CO2 consumption, etc. Also, fuzzy neural network model can be improved to receive crisp output instead of fuzzy output. Moreover, these models can be applied on the bigger dataset and on the other cities of Kazakhstan. Such improvement will be observed in future research.
References:
- C. Garcıa and O.Sanchez Meneses . The Fuzzy Nature of Climate Change Scenarios Maps. Technical report, 2014.
- C. Jianxian et al. An Air Quality Prediction Model Based on a Noise Re- duction Self-Coding Deep Network 15th May of 2020.
- Y. Ruiyun et al. RAQ–A Random Forest Approach for Predicting Air Quality in Urban Sensing Systems 2016.
- A. Kerimray et al. Spatiotemporal Variations and Contributing Factors of Air Pollutants in Almaty, Kazakhstan. Aerosol Air Qual. Res. 20: 1340–1352. https://doi.org/10.4209/aaqr.2019.09.0464, 2020.
- K. Golokhvast et al. Emissions from vehicles and human ecology Vladivos tok, 2016.
- Masih A. Machine learning algorithms in air quality modeling. Global Journal of Environmental Science and Management. – 2019. – . 5. – №. 4. – . 515-534.
- Cherkassky V. and Yunqian M. Practical selection of SVM parameters and noise estimation for SVM regression Neural networks 17.1, p.113-126, 2004.
- Chen, S. M. Forecasting enrollments based on fuzzy time series. Fuzzy Sets and Systems, vol. 81, pp. 311–319, 1996.
- Airkaz independent monitoring network, 2017. Retrieved from https://www.airkaz.org/. Accessed 15 December 2021