TWO DISCRETE EQUIVALENTS OF THE EDGEWORTH-WELDON-PEARSON INDEPENDENT HYPOTHESIS TEST
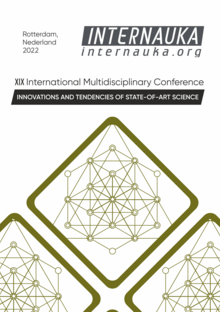
TWO DISCRETE EQUIVALENTS OF THE EDGEWORTH-WELDON-PEARSON INDEPENDENT HYPOTHESIS TEST
Julia Serikova
ORCID: 0000-0002-4959-321Х, engineer-programmer of 1st category, Joint-stock company «Scientific production enterprise «Rubin»,
Russia, Penza
An important indicator of the joint distribution of two empirical quantities is the correlation moment. It is defined as the mathematical expectation of the product of deviations of random variables. If the correlation moment is positive, then with an increase in the value of one of the empirical quantities, the value of the second tends to increase, and if the correlation moment is negative, it tends to decrease. However, in correlation analysis, one cannot judge the relationship between two empirical quantities only by the value of the correlation moment, since it depends on their dispersions. Therefore, in order to normalize the correlation moment, in the 1890s of the 19th century, a team of scientists Francis Edgeworth and Raphael Weldon led by Karl Pearson, introduced a correlation criterion:
(1)
The closer this indicator for two values is to the value of -1, the less they correlate with each other, otherwise, the values are more strongly correlated with each other. Formula (1) offers a convenient algorithm for numerical calculation of sample correlations in one pass. But sometimes this algorithm, for some sample values, can be computationally unstable. This situation is observed when evaluating small samples of biometric data [1]. This problem can be solved by using the synthesis of several statistical criteria [2].
Each statistical criterion can be assigned an equivalent artificial neuron. Consider, using the example of a centered and normalized sample of 200 experiments, two equivalent neurons: with linear quantizes (left side of figure 1) and two elliptical quantizes (right side of figure 1).
Figure 1. Neuron with linear quantizes and neuron with two elliptic quantizes
The software implementation of the first discrete equivalent of formula (1) with a spectrum of lines arranged with a step of ∆rd=0.01, as well as the results of a numerical experiment in MathCAD, are presented in figure 2.
Figure 2. First discrete equivalent Edgeworth-Weldon-Pearson (step: ∆rd=0.01)
It follows from figure 2 that a change in the size of the processed sample leads to a change in the step of the lines of the output spectrum, namely, when the sample is dragged, there is a clear decrease in the uniformity of the step between its lines. The resulting discrete analogue has a strong correlation with formula (1).
For normalization, you can carry out operations to find the minimum and maximum values of data variations. In this case, the correlation coefficients will be zero or close to zero. The software implementation of the second discrete equivalent of formula (1) with the spectrum of lines arranged with a step mmrd=1.00, as well as the results of the numerical experiment in MathCAD, are presented in figure 3.
Figure 3. Second discrete equivalent Edgeworth-Weldon-Pearson (step: mmrd=1.00)
Conclusion. Obtained two discrete equivalents of the Edgeworth-Weldon-Pearson Independent Hypothesis Test: strongly correlated (when counting the number of analyzed samples that fall into one of the four quadrants of the Cartesian coordinate system) and weakly correlated (when counting the number of combinations of analyzed samples that fall into each quadrant of the Cartesian coordinate system) with a continuum analog (1).
References:
- Serikova Yu.I. Multi-criteria neural network estimation of correlation coefficients for processing small samples of biometric data/ Yu.I. Serikova, A.I. Ivanov, T.A. Zolotareva, S.A. Polkovnikova // News of higher educational institutions. Volga region. Technical sciences.–Penza: Publishing House of PGU, 2021. No.1.P.13-22.
- Ivanov A.I., Bannykh A.G., Serikova Yu.I. Accounting for the effect of correlations by modulo averaging as part of neural network integration of statistical tests for small samples. Dependability. 2020. No.20(2). P.28-34. https://doi.org/10.21683/1729-2646-2020-20-2-28-34